In 2003, Floridians collected 23 Burmese pythons from Everglades National Park. The huge snake, they knew, didn’t belong there — it is native to Southeast Asia, though popular as a pet in the US. In 2005, the park found another 95. The following year, with pythons devouring native wildlife at a worrying rate, the South Florida Water Management District asked the US Fish and Wildlife Service to ban the invasive species from trade. The agency took until 2012 to do so, and that was probably too late.
“By the time they decided they were going to try and ban the sale, it was well established and spreading,” says Reuben Keller, an ecologist at Loyola University Chicago. Researchers think the animal is now a permanent Everglades resident. And the python is just one of 4,300 invasive species in the US that together cause more than $120 billion in damage every year.
The most cost-effective way to deal with those destructive species is to keep them from getting established in the first place, researchers have found — which means that ecologists and wildlife officials need to be able to predict, early on, which foreign organisms have invasive potential. It’s a skill they have been building in recent decades. Some of their newer prediction tools offer quick estimates of risk, allowing managers to focus their time and effort on species most likely to pose problems in the future. Other approaches deploy machine learning to recognize threats that other methods might miss, especially in cases where data are limited. Both strategies could help make preemptive bans more common — if the US could muster the political will to use them and act on them.
Block that pet!
Proactive bans are particularly useful for species that people want to introduce as pets, ornamental plants or game for hunting or fishing. Since 1900, the Fish and Wildlife Service has had the power to ban a species from importation, under legislation called the Lacey Act, if its potential environmental impact outweighs its value in trade. But to make this call, ecologists need to know ahead of time how much harm a species might do if introduced.
Until recently, the standard approach has been to dive deep into an organism’s natural history. Before banning Burmese pythons and other constrictors, for example, reptile experts had to learn all they could about each species — the kind of habitat it needs, what it eats and if and where it has become invasive before — then compile the results into reports so thick they “bordered on being books,” Keller says. Such a process takes months or years for each species, Keller and his colleagues point out in the 2016 Annual Review of Environment and Resources, which is one reason the constrictor ban came too late to prevent serious environmental harm.
This table, put together by authors of a 2016 article in the Annual Review of Environment and Resources, outlines the research, policy goals and management tools needed to prevent invasive species from establishing themselves and causing harm in new habitats.
Matters improved somewhat after 2010, when the Fish and Wildlife Service introduced a new invasivity prediction technique that was faster and less data-hungry. Called the Ecological Risk Screening Summaries, it catalogs the impacts of past introductions of a species and runs a climate matching model that scans for other environments in the US that the species could find homey. In less than two days, it labels a species with a high, low or uncertain risk of invasivity and highlights the regions where it might thrive best.
Kate Wyman-Grothem, who manages the program, says the speed sometimes allows her department to rapidly offer wildlife officials a quick assessment, pro or con, about a foreign plant or animal they’re worried about. But more often — about 80 percent of the time — species wind up in the “uncertain” category, because biologists can’t find enough published data to make a call.
Keller and other researchers thought they saw a way ahead. Around the same time that Fish and Wildlife produced its new tool, they began designing different prediction techniques that cope better with limited data. These methods use machine learning to analyze species that have and haven’t become invasive in the past and so identify key traits that determine invasivity. From that, researchers can predict which newcomers might follow the same path. The database “has now come to the size where we can at least say with more confidence what's likely to happen,” says Julian Olden, a conservation ecologist at the University of Washington.
Olden and his colleagues used this kind of machine learning to assess the potential invasivity of hundreds of fish species that might one day reach the Great Lakes as bait, food or escaped aquarium stock. They began by collecting data on 18 traits, such as salinity tolerance, number of offspring and breeding frequency, for 24 introduced species with a documented history in the ecosystem. Half were considered invasive to those lakes, while the other half had never become a problem. By contrasting the traits of the invasive species with traits of the innocuous ones, their machine-learning algorithm learned to correctly categorize each species more than three-quarters of the time.
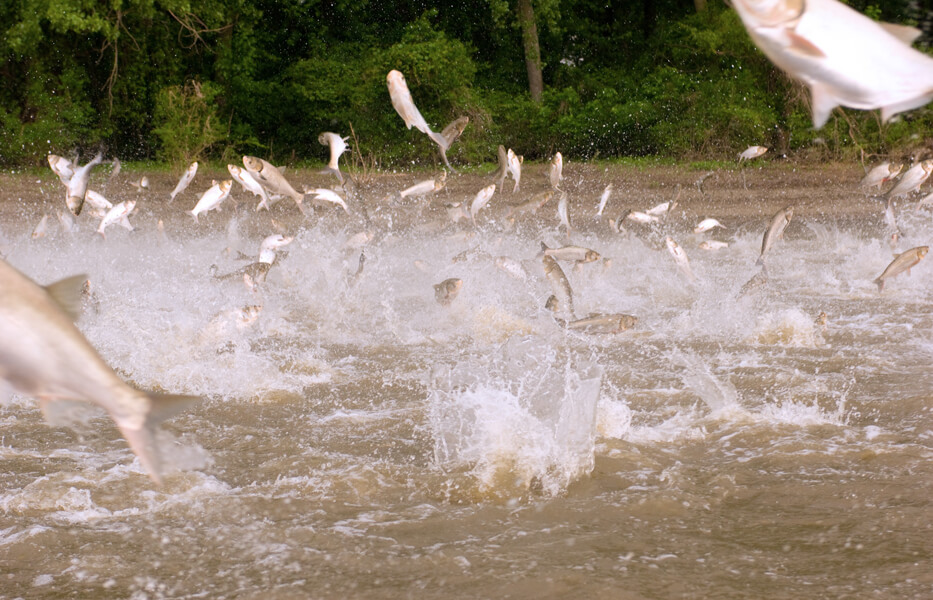
Invasive Asian carp leap out of the waters of the Illinois River near Havana, Illinois. Scientists fear these foreign fish, introduced to the US in the 1970s, will find their way to the Great Lakes, causing harm to native species and economic damage to fisheries.
CREDIT: JASONLINDSEY.COM
Then the scientists turned to 787 species of potentially invasive fish and used their algorithm to predict which group — invasive or benign — each species was likely to fall into. The team identified four strong threats to the Great Lakes: European catfish, blue catfish, striped bass and ide. All four, according to their model, are almost certainly going to become invasive if introduced to those waters, and all are likely to cause considerable damage, experienced ecologists think.
Difficulty doing the math
But the mathematics underlying machine learning — the advanced calculations that turn data points into perceived risk — are hard to understand. “In some cases, you can’t really explain what's going on with these tools,” says Keller, who worked with Olden on the project. Explaining the complex algorithms can pose a challenge when researchers try to persuade regulators, who may not be familiar with this type of mathematical model, to use them as a tool in decision-making.
Instead, for now these predictions play a background role in managing invasive species. The Nature Conservancy, for example, used them to help state and provincial officials in the Great Lakes region draft a list of the “least wanted” invasive species in 2013, says Lindsay Chadderton, aquatic invasive species director for the organization’s Great Lakes Program. The list included species such as killer shrimp and various water plants. Other US agencies, such as the Department of Agriculture’s Animal and Plant Health Inspection Service, use similarly intricate models to track where existing invasive species might roam next, as they did in 2015 when evaluating where feral pigs were likely to spread. Intricate models of scenarios can even highlight which habitats are, if not invasion-friendly, potentially good enough to keep a species alive, as recent research found with Asian carp in the Great Lakes.
This US map shows where feral pigs are most likely to be, or spread to, in the US, based on actual pig sightings made from 1982 to 2012 (areas enclosed by black lines), and assessment of environmental factors such as temperature extremes, forage and water availability, forest cover and habitat variety. In the scientists’ analysis, cold temperatures and poor water availability severely affected the pigs’ range. The most hospitable habitats appeared to be those that were ecologically varied, since they were more likely to supply all the needed resources.
Other nations have begun using a variety of predictive techniques to screen potential invaders before they can be considered for import. Decades ago, Australia and New Zealand made invasive species risk assessments integral to ecosystem protection. The nations have published a list of pets that are allowed for import, and any species not on the list is banned. Similarly, any ornamental plant not already on the approved list must be assessed for invasive potential ahead of time.
But the US still uses the Lacey Act just as it was written in 1900, which puts the burden of proof on those who seek to ban a species from trade. That requires a huge amount of evidence, a lot of time and more transparency than machine learning affords — with the result that proposed bans take an average of more than four years to implement. For a thriving invasive creature like the Burmese python, which eats virtually any animal it comes across and can lay 100 eggs at one go, that is plenty of time to get a foothold in a new environment.
“It’s glacial,” says Keller, “when you compare it to the speed at which these organisms can be released and then spread.” But until the US takes a more proactive, precautionary stance on imports of foreign species, predictive efforts are unlikely to be effective and bans will be too little, too late.