It’s been a long year, but a vaccine against Covid-19 has started to roll out across the United States. There won’t be enough to vaccinate everyone right away, so public health officials will need to figure out how to manage the slow ramp-up of immunity. Do we make faster progress by focusing on locked-down regions first, or on those that have tried to stay open? Dense urban areas or suburbs full of commuters? Should we skip over pockets with high levels of vaccine skepticism or push extra-hard to vaccinate there? And, ultimately, when will it be safe to relax, unmask and start hugging people again?
Those are difficult questions to answer, because the outcome depends on so many interconnected factors, including the unpredictability of individual humans’ minds and behavior. To make things even more challenging, human behavior is a moving target: What our leaders and friends say and do today influences what we ourselves do tomorrow. Effects can loop back to alter causes in a complex tangle that’s almost impossible for analysts to think through.
The real world is full of complex problems like this, from epidemiology to economics to foreign policy. But an emerging approach within the field of complexity science promises a better way to understand how the idiosyncratic, often irrational actions of many individuals generate complex collective outcomes. Instead of trying to analyze how a society functions from the top down, the new technique known as agent-based modeling tackles the problem from the other end, by focusing on the individuals themselves.
Like SimCity or the Matrix, agent-based modeling involves the creation of a computer-simulated world replete with thousands or even millions of individual people — the “agents” the name refers to — complete with homes, jobs, friends and realistic individual foibles. “Agent-based models are artificial societies of software people,” says Joshua Epstein, a computational social scientist at New York University who is one of the pioneers of the field. “These people behave like humans behave. They’re not perfectly rational or perfectly informed.”
When enough of these agents have been created, modelers turn them loose to move, act and interact. Just as the simple behavioral choices of individual fish cause them to collectively coalesce into a school, the cumulative effect of the agents’ individual decisions and actions leads to collective patterns such as epidemics, recessions or revolutions. Such models give analysts a way to explore the sometimes surprising outcomes that result from different policy options.
Already, agent-based models have taken over fields as disparate as traffic planning, securities trading and war gaming, and their use is growing in economics and political science. And within epidemiology, such models are yielding fresh insights into the way epidemics spread and evolve, what public health officials can do to control the outcome and the likely economic consequences of the pandemic. “It’s fundamentally changed the way people do infectious disease modeling,” says Epstein.
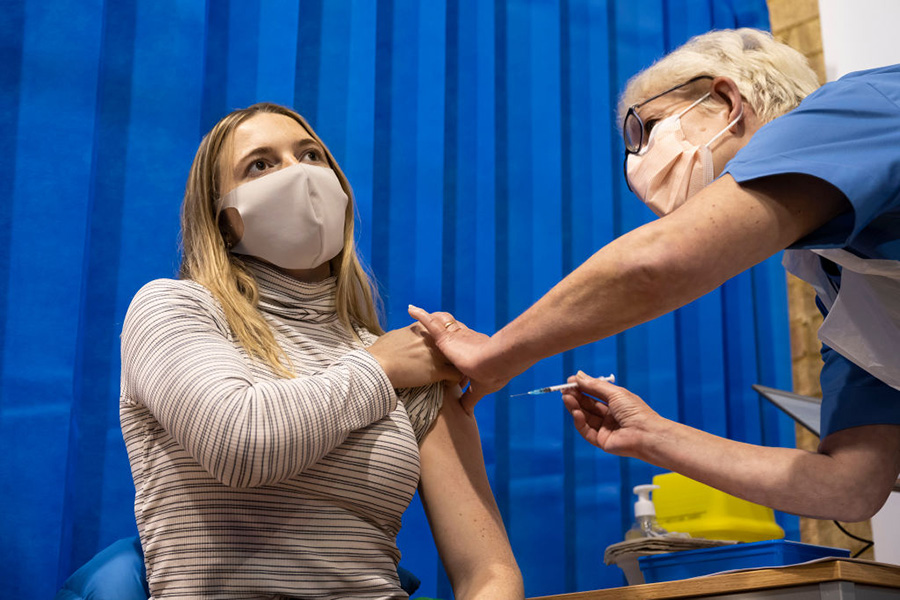
There won’t be enough Covid-19 vaccine to inoculate everyone right away. Agent-based models can help officials decide how to allocate their limited supplies.
CREDIT: MATTHEW HORWOOD / GETTY IMAGES
The conventional approach to disease forecasting doesn’t look at the behavior of individuals. Instead, epidemiologists typically sort the population into groups depending on, say, their virus status. These SEIR models (for susceptible, exposed, infectious and recovered, though many models subdivide further by age or other factors) assume that people encounter one another at random — the equivalent, as one researcher puts it, of pretending that people go home to a different family every night.
The real world has more spatial structure than that, of course. “It’s silly to have a model where I’m equally likely to have contact with a meatpacker in Kansas as [with] my neighbor across the street,” says Ian Lustick, a political scientist at the University of Pennsylvania who uses agent-based models extensively.
But until the advent of GPS-enabled cell phones and social media, researchers didn’t have enough data on how individuals move and interact during their daily lives to look at such granular issues. That has changed, and at the same time, computers have grown powerful enough to keep track of the location, interactions and attributes of millions of virtual people simultaneously.
Setting up an agent-based model is simple in concept but daunting in practice. The first step is to create a population of software people that resembles the real population being studied. For several research groups, this means agent-based models that span the entire country.
“We have every person in the US, where they live, where they go to school, where they go to work,” says Donald Burke, an epidemiologist at the University of Pittsburgh who helped develop one such model, known as FRED. Rather than copying reality exactly, though, FRED mimics its broad patterns. “There isn’t a Don Burke living at this exact address,” Burke explains. “But there is a person in their 70s living in a two-person household, with my income, in my census block.” And that not-quite-Don-Burke goes to work not at Pitt, but at a nameless employer of a similar size whose employees commute from similar distances. (Other groups have developed similar models.)
Once they’ve created the basic model, researchers can use it as a platform to address whatever specific question they need to answer. For pandemic-related questions, they specify realistic probabilities, based on available data, that each cyber-person will do things like wear a mask or refuse a vaccine, given their age, ethnicity and location. Then they turn their agents loose to go to work (at office or home), shop, meet friends and carry out all the other activities of daily life.
“I call that putting agents in motion. You have to make the city come alive, because that’s where the action is,” says Madhav Marathe of the Biocomplexity Institute at the University of Virginia (UVA), who heads a team running an agent-based model of that state’s 8-million-plus residents. As the agents live their cyber-lives, some will spread the virus to the people they contact, according to specified probabilities. After a few simulated weeks or months, the modelers can count up infections, hospitalizations and deaths to track the progress of the pandemic.
This approach not only offers predictions of the pandemic’s future trajectory, it also can give insights into the effects of varying public health strategies. For example, Burke’s team has modeled how the state of Iowa could best allocate a limited number of vaccine doses in order to slow the spread of the virus most efficiently. Health officials would need to vaccinate more aggressively in more densely populated parts of the state, they found, to counteract the natural tendency of the virus to spread more quickly where there are more people. Their model gives specific recommendations for how many vaccine doses should go to each medical center in the state.
Agent-based models can help forecast how much vaccine each region will need, based on differences in population density, people’s behaviors and other factors. Here, a model of Iowa, broken up into census tracts, shows where the virus continues to spread (shown in orange) and where it is under control (blue) as more and more people are vaccinated. Even when 80 percent of the population has been vaccinated, pockets of viral spread are likely to persist — areas that may need a stronger push for vaccination.
CREDIT: EPISTEMIX
Meanwhile, modelers at UVA’s Biocomplexity Institute have found that the best way to prevent spread among older people most vulnerable to Covid-19 may not be to vaccinate them first, as many states are planning. “If you take a fixed amount of vaccine, you actually end up saving more lives in the older people by giving it to the children, because you’re breaking the chain of transmission,” says Bryan Lewis, a computational epidemiologist at the institute.
The UVA team had also advised the university on its best strategy for reopening to students this fall. The model highlighted a surprising result: How many students the university could allow on campus depended critically on how many isolation rooms the university could provide for students who tested positive for the coronavirus, says Chris Barrett, a leader at UVA’s Biocomplexity Institute who was among the first modelers to adopt agent-based approaches back in the 1980s.
To make their models more realistic, some researchers are adding further layers of psychological realism. Epstein’s team at NYU, for example, is now taking into account how fear of Covid-19 affects people’s behavior. Last spring, as the pandemic was just taking hold, fear of the virus spread rapidly — a different sort of contagion, Epstein says. Driven by that fear, states shuttered their economies and people sheltered at home. That slowed the spread of the virus dramatically, but then as complacency replaced fear, people relaxed.
By tracking how fear and complacency spread from person to person, Epstein’s team has shown that complacency can trigger a fresh wave of virus infections when society opens up again. “When people emerge, you’re pouring susceptible fuel on the infected embers, and you get second waves,” he says. “That’s exactly what we’re seeing now.” Couple that with a second fear — of the vaccine itself — and things get messy. “You have multiple dynamics going on, which can produce very complicated trajectories, including multiple waves even after a vaccine is introduced,” Epstein says. Agent-based models may be the best way to sort out such complexity. He and his colleagues are now exploring whether they can “take the temperature” of fear and complacency in the wording of people’s Twitter posts to give an early warning of future waves of infection.
At UVA, psychologist Mark Orr is taking a similar approach with their agent-based model, trying to capture how people decide about behaviors such as mask-wearing based on information coming from the media, government, friends, family and neighbors. The work has just begun, but already his team is exploring ways to predict people’s mask-wearing behavior from their social media feeds. By quantifying some of the social factors that affect that decision and by understanding the psychology of the decision-making process, Orr hopes to be able to explore how interventions such as public health announcements or mask-wearing by prominent people can help shape the outcome.
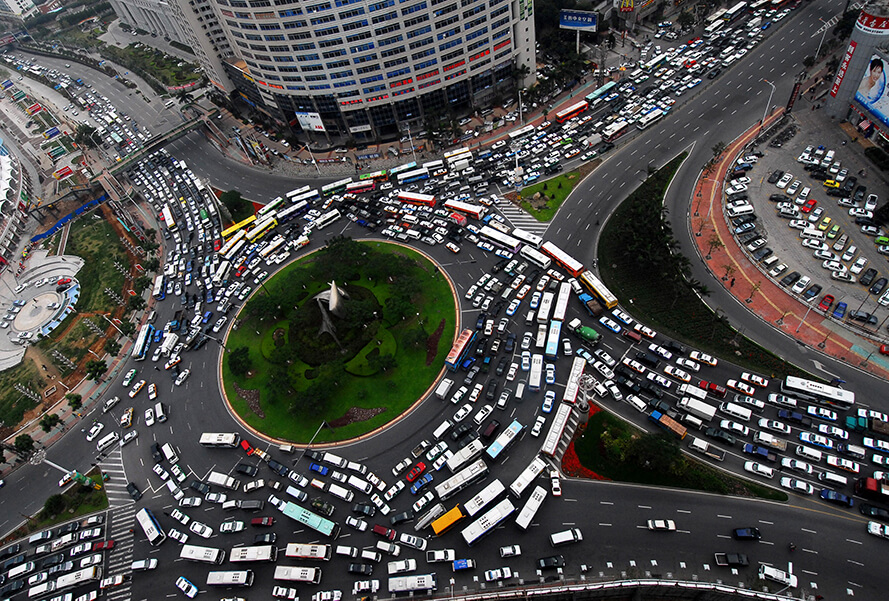
Most traffic planners now use agent-based models to analyze traffic flow to try to prevent snarls like this one in Xiamen, China.
CREDIT: IMAGINECHINA LIMITED / ALAMY STOCK PHOTO
Visualizing the impact
Agent-based models don’t just offer novel insights. They also make it easier for analysts to communicate their results, because their focus on individuals makes immediate sense to policymakers and the public. “If you see a map with individual humans on it, and the epidemic spreads with movement of red dots across a map, that’s more understandable than seeing an equation or a graph,” says Burke.
Even more powerfully, the spatial detail of such models means that decision makers can visualize the impact of their choices on their own constituencies. After a measles outbreak at Disneyland in California, for example, state Sen. Richard Pan cosponsored a bill in 2015 to require measles vaccinations for all schoolchildren unless they had a medical exemption. Pan used Burke’s model to show other senators what a measles epidemic would look like in their hometowns with and without the measure. The bill passed, and immunization rates rose in California. “Sen. Pan has told us that having this tool was very important in conveying this idea,” says Burke.
Powerful as they are, agent-based models may not be the best tool for every job. Rather than sussing out the psychological underpinnings of individual decisions and then programming them into the rules for agents, for example, it may sometimes be quicker and just as useful to simply throw real people into a room to work through the problem in a game (see Gaming for disaster).
Other times, a simpler analysis may yield the kind of answer policymakers need. Consider the question of whether public health officials are adequately prepared for a future pandemic. The answer may not depend on details of who contacts whom, says Edward Kaplan, an operations researcher at Yale University. “My question is, what is the worst case? If I can handle that, I can handle the others,” he says. And that worst-case scenario depends more on how infectious the pathogen is and how quickly public health officials can test and isolate infected people, not the details of individuals’ contacts, says Kaplan.
Agent-based models also demand large amounts of data and vast computing resources — often hours of supercomputing center time per run. That can make them cumbersome to use, especially when policymakers need answers quickly to urgent questions, Kaplan says.
And there’s another drawback to agent-based models: Their relative newness in the field means that modelers have not yet settled on best practices for building the models, or standard procedures for recognizing when a model is sound. “I think this is one area where we’ve lagged behind a little bit — in coming up with robust validation techniques,” says Melissa Tracy, an epidemiologist at the University at Albany School of Public Health, who coauthored a review of agent-based models in public health in the Annual Review of Public Health. Researchers are working on the validation problem. However, she notes, “it’s still in the early stages — and it’s a more difficult validation problem than more simple types of analysis.”
Despite these limitations, agent-based models have already taken over several fields, says Robert Axtell, a computational social scientist at George Mason University. Traffic planners, who once designed road networks and synchronized traffic signals by modeling vehicle flows like the flow of liquid in a pipe, now almost always use the agent-based approach, which explains jams as the collective effect of the behavior of many individual drivers. Hedge funds and other major securities traders, too, now use agent-based analyses of who else is trading a given security on a given day to forecast supply and demand. And the US military, Axtell says, is now the largest user of agent-based models in the world, simulating the actions of individual soldiers on the battlefield to predict everything from logistics to outcomes of battles. These three fields, together with the emergence of its use in epidemiology, make up what Axtell likes to call the “three-and-a-half successes of agent-based models.”
They are paying off in other fields, too. At UVA, modeler Abhijin Adiga has simulated the movements of a serious pest of tomatoes, the introduced moth Tuta absoluta, in Southeast Asia. Instead of individuals, the “agents” of Adiga’s model are grid squares on a map, but his simulation accounts for both the moth’s flight from one square to an adjacent one and also longer-distance dispersal as moths hitch rides on tomatoes moving to market. Whereas conventional pest-risk maps merely identify suitable habitat for the pest, Adiga’s simulations have allowed him to identify key choke points for the pest’s dispersal — often at wholesale markets — where cash-strapped governments can best allocate their limited resources to control the pest.
Agent-based models of the tomato leafminer in Thailand show the possible benefit of restricting tomato shipments from areas where the pest is common. On the left, a map shows how the pest’s range spreads from its initial locality (upper left, darkest blue) both locally and in leaps to other cities (center and bottom) in the absence of restrictions. On the right, the map shows that restricting tomato shipments from the leafminer’s original locality keeps the pest outbreak confined to that area and prevents long-range leaps.
More ambitiously, Axtell has built a representation of all 6 million US firms with one or more employees. The firms in Axtell’s simulated economy mimic the sizes and interactions of real firms, but without depicting actual companies in every detail: There is no Ford or Exxon, but there are nameless firms with their general characteristics.
With his model, Axtell can now explore questions like the effect of laying off 8 million workers in a few weeks during the pandemic lockdown, either spread randomly across the economy or concentrated in specific industries like restaurants. He can also generate finer-scale economic data than are generally available in real life. Until recently, for example, the US government reported the number of new firms that form each quarter — a number that changes little from quarter to quarter, suggesting a relatively stable economy. But when Axtell looked on finer time scales, he found a totally different picture. “When you look at the data on a weekly basis, you say, holy moly, there’s so much flux!” he says.
Meanwhile, Lustick is applying the technique to study political instability in countries around the world. The agents in his simulation are influential people in the society, together with their many connections through friendship, kinship, ethnic and religious affiliations, and other links. As the agents interact, the model tracks the shifting political fortunes of the various factions in the society, and suggests which future outcomes are most likely.
That’s different from predicting what will happen, Lustick is quick to caution. “You’re not making a point prediction,” he says. “You’re generating distributions of probabilities.” That can still be useful as a guide to what policies are likely to work best. If a particular policy under consideration never leads to the desired outcome, planners can drop that idea. Analyses after the Syrian government committed atrocities against its own people in 2012–13, for example, showed that the policy of continued diplomacy adopted by the US government was the only one that had any chance of preventing the atrocities — even though, in fact, it did not. “Just because you don’t get the outcome you want doesn’t mean you didn’t do the right thing,” says Lustick.
As computing power continues to grow, Axtell foresees the day when agent-based models get powerful enough to take on big questions like climate change and social justice, which are also the emergent results of millions of individual decisions. “It seems like a natural thing to use agent-based models for climate change, because there’s a lot of heterogeneity, and that’s something they do well,” he says. “My guess is that the Intergovernmental Panel on Climate Change report for 2050 will be based on an agent-based model of the whole world.”
Someday, Lustick says, we may all carry around an agent-based model of the world, running continuously on our phones. With it, we could manage everything that’s important in our lives, from economic trends to investment decisions to where our kids are likely to be today or tomorrow. It’s not quite the Matrix, but it’s close.
This article is part of Reset: The Science of Crisis & Recovery, an ongoing series exploring how the world is navigating the coronavirus pandemic, its consequences and the way forward. Reset is supported by a grant from the Alfred P. Sloan Foundation.