Every year, shortly after the Super Bowl, America’s best college football players head to Indianapolis. It’s a rite of spring, like the migration of birds. Their destination is the Combine, a weeklong event where National Football League teams evaluate the talent to determine whom they’ll select during the upcoming NFL draft.
In a convention center ballroom not far from the stadium, another “combine” is taking place. Here the marquee event is not the 40-yard dash but the six-minute research presentation. The competitors are not sports stars but data scientists who’ve come for the final round of the Big Data Bowl. Launched by the NFL in 2018, this competition challenges teams of researchers to apply analytics and AI tools to football data.
Over the last several years, analytics have enabled NFL teams to evaluate players in ways not possible before — for example, assessing a defender’s ability to create tackling opportunities, not just completed tackles. Coaches use the metrics to streamline game preparation. And fans, as well as bettors and bookmakers, crave the insights offered by what the NFL calls Next Gen Stats.
Big Data Bowl competitors, like their player counterparts, can be picked up by a football team. About 40 have been hired by some 20 teams, says Mike Lopez, the NFL’s senior director of football data and analytics. Others have joined companies, including Zelus Analytics, StatsBomb and Telemetry Sports, that provide data and services to NFL teams and other sports teams. (Stephanie Kovalchik, a data scientist at Zelus Analytics, described how the same techniques can be applied across different sports in 2023 in the Annual Review of Statistics and Its Application.)
More than 300 entries in 2024’s Big Data Bowl were winnowed to five finalist teams invited to Indianapolis. “You have academics here, industry professionals, students, and collaborations between students and coaches,” says Ron Yurko, a statistician at Carnegie Mellon University in Pittsburgh and one of this year’s finalists. The goal is to gain insight “that has football meaning.”
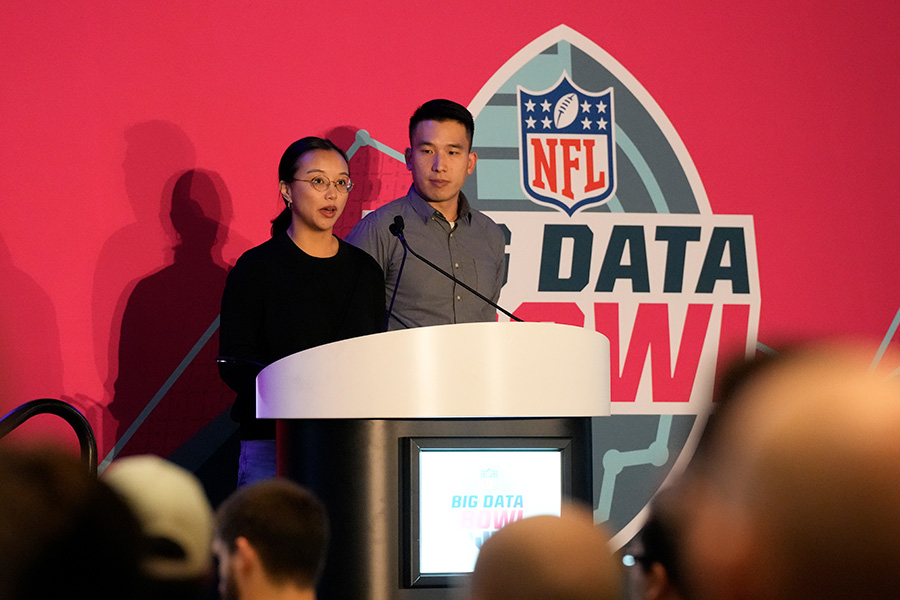
Data scientists Katherine Dai and Matt Chang explain their prizewinning research on missed tackle opportunities at the 2024 Big Data Bowl.
CREDIT: AP IMAGES FOR THE NFL. USED WITH PERMISSION
Tracking every move
Beginning in 2014, NFL players have worn a computer chip in their shoulder pads. Ten times every second, the chip records player location, direction, velocity and acceleration. “Next Gen Stats in football means player tracking,” Lopez says. Since 2017, a similar chip has been in the ball, and all of the data have been made available to all of the teams since 2018.
But that’s only part of it. What really separates today’s statistics is the way they are analyzed. The goal is to understand not just what happened, but also why. Why did this run gain only three yards, while that one went for 88 yards and a touchdown? In the process, Next Gen Stats for the first time can quantify the contributions of the unsung players who don’t ever touch the ball, such as the blocker who sprang the runner loose for that 88-yard touchdown.
Katherine Dai, one of this year’s finalists, says the research presented in the 2024 Big Data Bowl featured two complementary approaches. Analytics generally use human-derived formulas to extract meaningful metrics from the data. In contrast, machine learning — the approach that has brought us generative AI like ChatGPT — trains the computer to figure out the most predictive features.
If a metric just captures what happened, it’s probably analytics. If it relies on a prediction or a probability of what could have happened, it’s probably machine learning, Dai says.
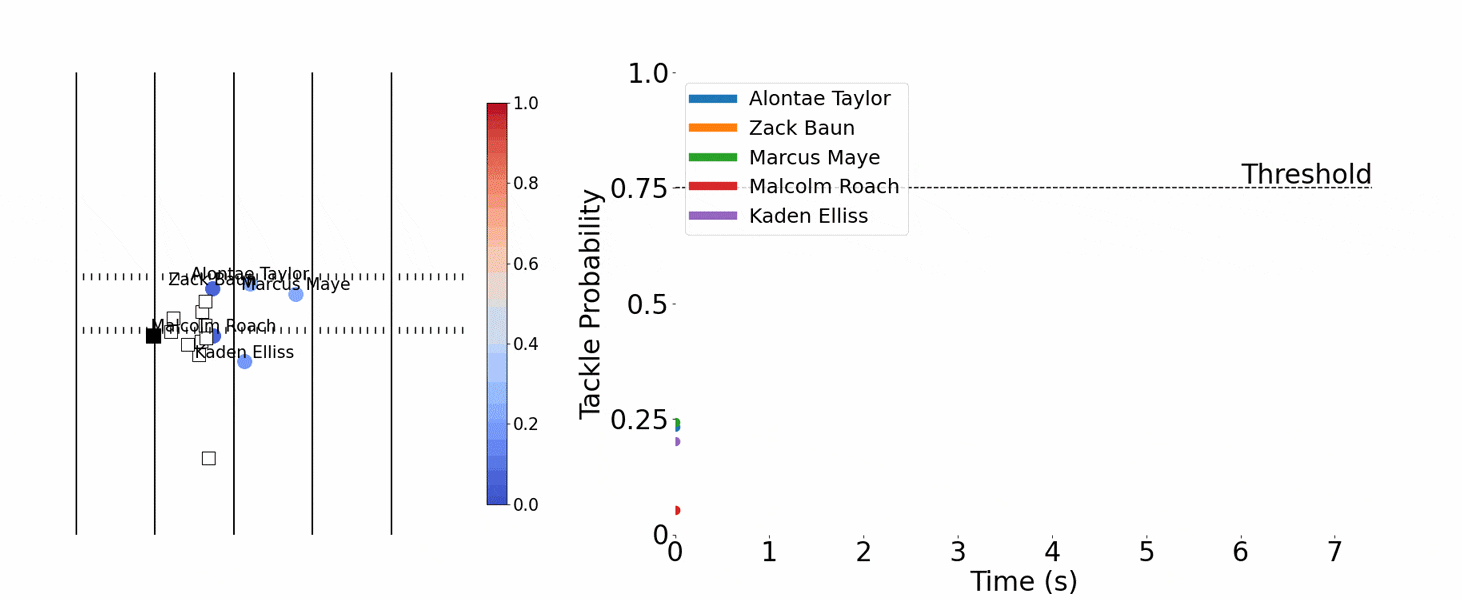
The winning entry in the 2024 Big Data Bowl assigns a missed tackle to a defender when his probability of making a tackle rises above the 75 percent threshold for at least half a second before dropping back and neither he nor a teammate makes the tackle in the next second. This example, from a November 7, 2022, game between the Baltimore Ravens and the New Orleans Saints, shows the paths of five defenders (red squares on the left are missed tackles) and the runner, Kenyan Drake (black square). Alontae Taylor, Kaden Elliss, Malcolm Roach and Zack Baun all had a chance to tackle the runner (right graph) before Marcus Maye succeeded.
CREDIT: COURTESY OF KATHERINE DAI
When the NFL hired Lopez, a former statistics professor at Skidmore College in New York State and a former college football player, he sold them on the idea of the Big Data Bowl in his interview and promised that, as in the 1989 film Field of Dreams, “if you put the data out there, the analysts will come.” But three hours before the submissions deadline for the first competition, only three had come in, and he was getting nervous. “Then they started pouring in,” he says — 100 between 9 p.m. and midnight. “That was a lesson to me on how data scientists work.”
Every year since, the competition has had a specific theme. In 2020, for example, tracking data were used to predict the expected yards gained by a running play at any instant during the play, based on locations of the 22 players and their speeds — a task made to order for machine learning.
The winners were a pair of data scientists based in Austria, Philipp Singer and Dmitry Gordeev, who had only rudimentary knowledge of American football. They were both “grandmasters” of computer competitions, and they developed a neural network, a common type of machine-learning algorithm, that blew the others away.
Singer and Gordeev’s algorithm was adopted into several new Next Gen Stats: expected rushing yards, rushing yards over expected (the difference between actual yards gained and the prediction), first down probability and touchdown probability. The stats debuted on national TV just six months later.
Securing the win
If you were going to bet on the 2024 winner, a smart choice might have been Yurko’s team. He worked on football analytics even before the NFL got interested. In 2017, Yurko and colleagues presented a technique for estimating a football player’s WAR, or wins above replacement, defined as the number of fractional wins created by a given player compared with an average replacement player. (It’s “fractional” because only some portion of the credit for a win is granted to the player.)
In baseball, WAR has been a go-to metric for more than 20 years, but it wasn’t so easy to generalize to football. Yurko’s paper, reported in the Journal of Quantitative Analysis in Sports, inspired Nate Sterken, winner of the inaugural Big Data Bowl and now lead data scientist for the Cleveland Browns, to go into football analytics.
Yurko was a Big Data Bowl judge, but stopped judging when he joined the Carnegie Mellon faculty because, he says, “I wanted my students to win.” Indeed, his students were on two of this year’s five final teams, and one student, Quang Nguyen, was a finalist for the second year in a row.
The theme for 2024 was tackling, and Yurko’s team used tracking data to calculate a physics-based measure for fractional tackles. After identifying when the runner’s forward momentum decreases significantly, the computer identifies the nearby defenders and divides credit accordingly. If two defenders are nearby when the runner’s momentum decreases by 50 percent, for example, they each get credit for 25 percent of the ultimate tackle.
The fractional tackle metric highlights the contributions of defensive linemen, who often slow the runner down but less often complete the tackle. These linemen (or their agents) can use this stat when negotiating salaries, for instance.
But Yurko’s team didn’t win. Instead, victory — and a prize of $25,000 — went to Dai, Matthew Chang, Daniel Jiang and Harvey Cheng. Three of the data scientists had met as graduate students at Princeton. None had entered a coding competition before. “We joked that it would be a good excuse to watch some football,” Dai says. None had worked in sports analytics, but “we’re open to it,” she adds.
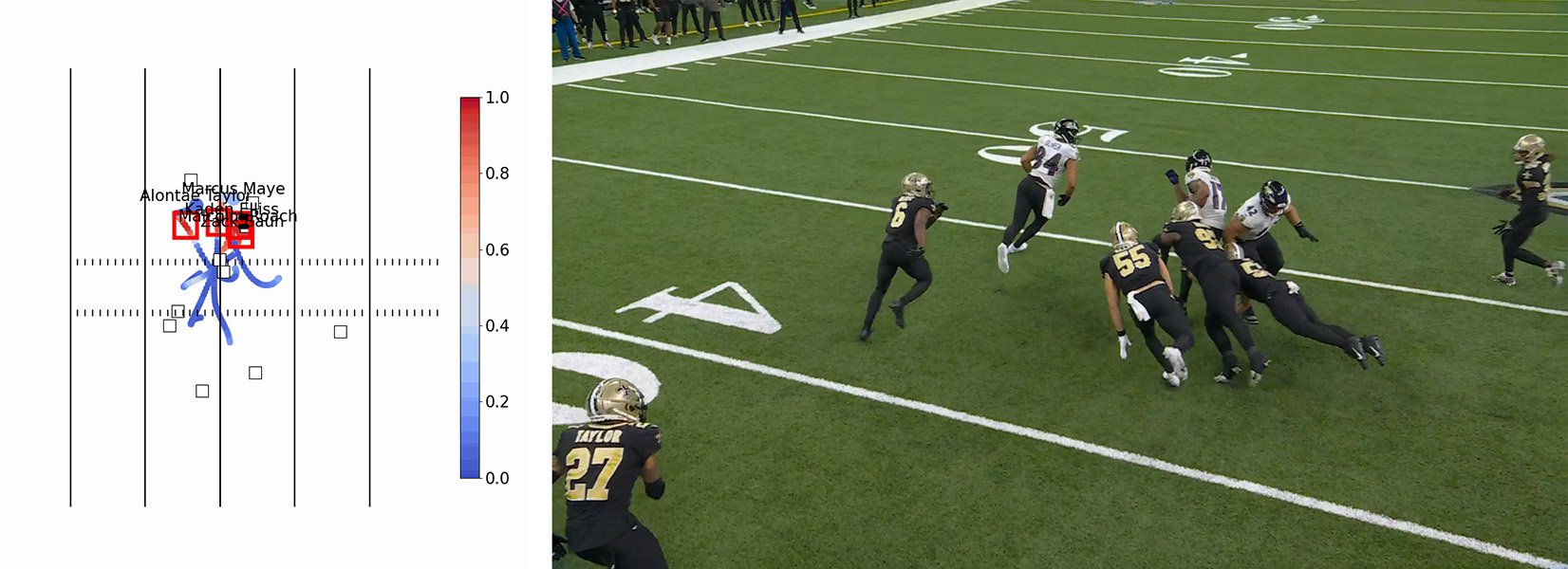
With 13:05 left to play in the November 7, 2022, game between the Baltimore Ravens and the New Orleans Saints (right), the ball carrier Kenyan Drake (number 17 in white) has broken free from four tackling opportunities (red boxes, left). A human scorer charged only Malcolm Roach (number 97) with a missed tackle, but advanced data analytics concluded that three additional players should also have been charged with missed tackles (27, 55 and 53, diving). Another player, Marcus Maye (6), caught up with Drake at around the 50-yard line to finally make the tackle.
CREDITS: LEFT, COURTESY OF KATHERINE DAI. RIGHT, NFL. USED WITH PERMISSION
The team first tried to predict at any moment the probability of a tackle within the next second, but three algorithms that used neural networks weren’t accurate enough. So the team pivoted to decision trees, another well-known machine learning method, and hit pay dirt. Predictions of tackles improved, plus the team could identify near misses.
After charting the probabilities of multiple defenders getting a tackle on the same play across time, Chang noticed peaks and valleys. Comparing that with video of the plays revealed that the peaks matched up with someone missing a tackle. “All credit to Matt,” Dai says.
That led the team to a quantifiable definition for a missed tackle: It occurs when a defensive player’s probability of making a tackle exceeds 75 percent for more than half a second, then drops below 75 percent, and neither he nor his teammates make a tackle within the next second. It’s a simple definition, but the trick is computing the probability, which depends on machine learning.
All of these metrics still have room to evolve. Matt Edwards, head of American football analysis at StatsBomb, notes that both teams evaluated tackling based on proximity to the runner, not actual contact. That’s a limitation of the tracking data; the chips can’t tell whether the players are touching. The old-fashioned approach of having humans watch game video can do that.
And though chip-based data aren’t available for college players, some teams will take tracking data from video alongside new analytics into consideration in the next NFL draft, which begins April 25.
Edwards points to the Los Angeles Rams. Instead of relying on how a player performs in the 40-yard dash and other Combine events that don’t replicate what happens in an actual game, the Rams are looking solely at tracking data. “You want to know how quickly he gets off the ball,” Edwards says. “What is his closing speed and reaction time when the ball is in the air? These are football-specific skills.”